Spring AI’s 0.8.0-SNAPSHOT release includes support for Image Generation using Dall-E 2/3 or Stability. This was added on January 24th and has not yet been documented but a video by Craig Walls describes how to use the new functionality.
I thought that an interesting example to try would be to combine ChatGPT with Dall-E. This way I can take a restricted range of parameters for an image (ie mood, animal, activity) and ask ChatGPT to expand this into a detailed prompt, which I can then use to generate an image. The idea here is to take user input for the prompt but to restrict what they can specify, maybe through some dropdowns. Another way of doung this would be to use ChatGPT to check freeform user input, but this seems to be simpler.
The example was pretty easy to put together. I used the org.springframework.ai.openai.OpenAiChatClient class to communicate with chatGPT followed by the org.springframework.ai.image.ImageClient class to generate the image using Dall-E 3. A simple Controller took some GET parameters and placed them into a prompt template:
I want to generate amusing images.
These images should feature an animal. The animal chosen is {animal}.
The animal in question should be {activity}.
The picture should make the user feel {mood}.
This template prompt could be changed to further restrict or specify the sort of image being produced through prompt engineering.
There’s a fair amount of Spring magic tying things together – in particular a @Configuration class that sets up the OpenAIImageClient, since auto-configuration is not yet available. The Controller method is as follows:
@GetMapping("safeimagegen")
public String restrictedImageGeneration(
@RequestParam(name = "animal") String animal,
@RequestParam(name = "activity") String activity,
@RequestParam(name = "mood") String mood) {
PromptTemplate promptTemplate = new PromptTemplate(imagePrompt);
Message message = promptTemplate.createMessage(Map.of("animal", animal, "activity", activity, "mood", mood));
Prompt prompt = new Prompt(List.of(message));
logger.info(prompt.toString());
ChatResponse response = chatClient.call(prompt);
String generatedImagePrompt = response.getResult().toString();
logger.info("AI responded: generatedImagePrompt);
ImageOptions imageOptions = ImageOptionsBuilder.builder().withModel("dall-e-3")
.build();
ImagePrompt imagePrompt = new ImagePrompt(generatedImagePrompt, imageOptions);
ImageResponse imageResponse = imageClient.call(imagePrompt);
String imageUrl = imageResponse.getResult().getOutput().getUrl();
return "redirect:"+imageUrl;
}
This is not a particularly sophisticated piece of code, but it does show how simple it is to get SpringAI examples working.
I submitted a request for a picture of an aligator rollerblading, and set the mood as “joyful”. ChatGPT then generated a detailed prompt:
The image features a cheerful green gator. He’s wearing a pair of shiny, multicolored rollerblades that sparkle as they catch the light. His eyes are wide with excitement, and his mouth is stretched in a wide, friendly grin, revealing his white teeth. He’s standing in a beautiful park with green trees and flowers in the background, and there’s a clear blue sky overhead. He’s waving at the viewer as if inviting them to join him in his rollerblading adventure, adding to the joyful and playful vibe of the image.
And then the browser was redirected to the image:
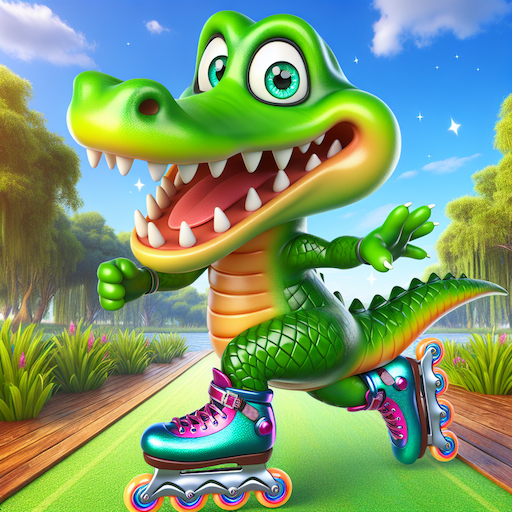